How Machine Learning Is Reinventing Predictive Analytics for Precision and Insights
Advances in machine learning are taking a new-age turn by redesigning how organizations make predictions and decisions. Having the possibility of recognizing patterns and allowing the forecast of future results accurately, the techniques of machine learning are pushing predictive analytics into a new high level. This powerful combination is in the middle of changing industries—finance to health—yielding clearer insights and smarter strategies.
Playing a very important role in predictive analytics, machine learning has the capacity to look through piles of data lighting fast. It helps the business and researchers find key trends which, when analyzed by any traditional method, prove to be very time-consuming. Using historical information, it provides companies with recommendations and risk assessments to better exploit and improve operational combativeness.
Any person who would like to enhance his or her analytical skills will need to understand the role of machine learning in this field. Companies are using data to make decisions, so being aware of how to use these advanced tools can make a big difference.
Key Takeaways
Machine learning enhances the accuracy of predictive analytics.
It detects trends housed in large data sets.
These technologies enhance decision-making.
Basics of Machine Learning for Predictive Analytics
Predictive analytics is what machine learning is at the core of. It helps one make accurate predictions regarding future outcomes through the assistance of data and algorithms. One cannot design predictive modeling effectively without having in-depth knowledge about the basics of machine learning.
Introduction to Machine Learning
It is a subset of artificial intelligence that enables the system to learn from data. It involves algorithms that can identify patterns and make decisions with little or no involvement of humans.
There are three major categories into which machine learning can be divided:
- Supervised Learning: The models are trained using labeled data, and the outcome is already known.
- Unsupervised Learning: It involves fitting models into unlabeled data to uncover hidden patterns. Reinforcement Learning: The models learn as feedback is provided in the form of rewards and penalties. Each of these types serves different purposes in predictive analytics.
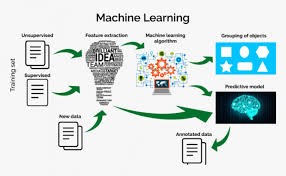
Predictive Modeling Techniques
Predictive modeling is concerned with the construction of models to predict future events. Common techniques include:
- Regression Analysis: It infers the continuous output on the basis of the input variables. This helps to understand what is happening between variables.
- Decision Trees: These are structures almost like the schematic flow and help to make decisions. They illustrate how the data breaks down into smaller subsets while incrementally developing an associated decision tree.
- Neural network: It works on mimicking the structure of the human brain in order to recognize complex patterns in data. They work really well on high-dimensional data, like images.
All of these methods have their own advantages, and the choice depends on data and objectives.
Data Preparation for Machine Learning
Effective data preparation is the key for success in making a model with machine learning. Good-quality data prevents poor prediction models. Several vital steps of this process are:
Data Cleaning: Eradicate errors, duplicates, and irrelevant information.
Feature Selection: Selection of Variables or features that are useful for prediction.
Data Transformation: Normalization or scaling of data to ensure features of data are on the same scale, thereby contributing equally to the model.
Proper data preparation is key to effective learning and reliable predictions; this makes this step so vital in the process of predictive analytics.
Applications of Machine Learning in Predictive Analytics
Machine learning supports predictive analytics in these areas of operations. It assists an organization to make informed decisions from an analysis dedicated to data patterns. Key applications include risk assessment, predictive customer behavior, operational efficiency, and market trend analysis.
Machine learning helps companies in assessing risks by crunching massive bits of data in the fastest way possible. It is the algorithm that identifies patterns within the historical data, which can flag potential risks. The classic example, within finance, is where models predict loan defaults given credit scores, financial history, and the state of the economy.
Claims are estimated using machine learning. By reviewing past claims, they can identify trends showing higher-risk areas. That enables them to set more correct premiums and hence reduce losses.
In healthcare, predictions are made by assessing the risk that a patient may have based on their medical and lifestyle history. Which means, hospitals focus more on patients who need care urgently, hence enhancing general outlook of their patients.
Predictive Models for Customer Behavior
Businesses use ML to decipher customer behavior. Algorithms, with the help of their purchase data, are able to predict trends of buying in the future. This consequently empowers focused marketing strategies.
E-commerce companies make use of browsing history and shopping patterns to suggest products to customers. This can prove to be a personal touch that helps to maximize sales and enhance customer satisfaction.
Retailers use machine learning for effective inventory optimization. Based upon the anticipated customer demand, it allows for adequate inventory. This significantly reduces cost and improves efficiency by ensuring the right products are available at the right time.
Operational Efficiency and Maintenance
Machine learning ensures operational efficiencies in a host of industries. For example, in manufacturing, there are predictive maintenance models that work through the analysis of data on equipment to foresee failures and prevent them. In general, this new approach minimizes downtime and also helps save costs.
For example, the sensors in the machines give out the temperature, vibrations, and the data about the machine usage patterns. So, in case of any inaccuracy in the machine that indicates wear out, the sensors trigger some alarm for the problem before it is completely broken. This, in using the machines to increase the productivity in this sector, improves the machines’ lifespan.
Routing for deliveries and the routes in logistics can be optimized with the use of machine learning; thus, the analysis of the traffic data and the weather pattern help in reducing the delivery time and reducing fuel costs. So, the companies would work efficiently and also please their respective customers.
Market Trend Analysis
Another way machine learning helps businesses is by staying competitive through the understanding of market trends. The developed algorithms scan through a great deal of market data to notice tendencies on the market. This way, it helps companies make strategic decisions.
An application is in financial firms, which analyze the information in social media and news articles to put into context, trying to estimate the market situation or public opinion. Such information in real time can be very important to predict stock movements and new investment opportunities.
Retailers such as Tesco use machine learning in analyzing consumer trends. When businesses are well aware of what products are in demand, that helps to change their offering. Such agility improves their market responsiveness and drives their sales growth.
Also Read :
- The Rise of Quantum Computing: What It Means for Technology
- The Future of Mobile Technology: Trends to Watch in 2024
- Blockchain Technology: Understanding Its Impact Beyond Cryptocurrencies
- Technology News
- Technology with Fidelity Global Technology