Understanding the Role of Data Science in Modern Businesses: Applications and Insights to Succeed
Modern businesses have adapted to working in accordance with the insights derived from data. The analysis of large data sets is used to gain insights that drive decisions made for increasing a company’s efficiency. Adopting data science helps businesses understand customer needs and thus optimize their processes, which in turn gives them an edge over others.
In the information age, that ability to interpret data and turn it into action sets one company apart from another. Data Science empowers organizations with correct decision-making capabilities, driving innovation, and bringing better products and services to the marketplace. Such is a new way to boost productivity, which paves the way for innovation across diverse industries.
With an ever-increasing volume of data, the reliance on data science will continue to surge. Through the adoption of these techniques, enterprises put themselves at a vantage position where long-term success and adaptability in an ever-fast-changing market become possible.
Key Takeaways
Data science helps businesses understand customer behavior.
It optimizes operations for improved efficiency.
It drives innovation and competitive advantage.
Fundamentals of Data Science in Business
Data science is part of the daily running of a business today. Organizations use it to make decisions that are informed by data and not driven by gut feel. This chapter touches on the broad strokes which define how data science is used within a business environment.
What is Data Science?
Data science is a blend of statistics, computer science, and domain knowledge. It deals with extracting meaningful information from large volumes of data. Extracting information from data consists of data collection, processing, analysis, and visualization.
Businesses make use of data science so as to discover patterns and trends. For example, analysis of customer behavior will aid in enhancing products. Predictive modeling is another tool to project future trends and to know what strategy to adapt.
Extraction of meaningful information from data is done by using tools such as Python or R programming languages by the data scientists. Also, they use SQL software for managing databases. Their work helps in making sense out of complex data.
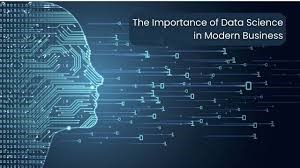
Evolution and Importance
The field of data science has undergone several changes in the past two decades. From earlier times, when companies used simple statistics to drive key decisions, technology has evolved and improved the capabilities of data.
Today, businesses create enormous volumes of data every day. This boom in data makes it fundamental for companies to harness it effectively. Organizations that adapt data science in their organizations drive efficiency, rich customer experiences, and improved revenues.
The edge is overwhelming when it comes to analyzing data in a competitive market. Any company that utilises strategies driven through data is better at quick adaptability to changes in the market, or to the needs of customers.
Types of Data Analytics
There are four principal types of data analytics used in business:
- Descriptive Analytics: This helps describe past data. Businesses use it to understand trends and performance over time.
- Diagnostic Analytics: This involves the determination of the causes of past outcomes. Through it, organizations are able to learn from past mistakes.
- Predictive Analytics: It involves the prediction of future events by making use of historical data. It is applied in marketing and finance.
- Prescriptive Analytics: This gives recommendations for actions to take based on the provided data. This helps in optimizing decisions and strategies.
Each of these has a special function and is important in providing insight for a business. Such types of analytics have enabled companies to leverage this data to make informed decisions and thereby be ahead of competition.
Application and Impact
Data science is an important player in how modern businesses run. Applications such as enhancing decision making, customer experiences, or even smoothing of operations can be achieved through it.
Business Intelligence and Decision Making
Data Science enables the translation of raw data into meaningful insights. In business, it is now possible to effectively analyze trends and patterns. Decisions are data-driven by tools such as dashboards and reports.
For instance, a company can be able to track the sales data across various regions. It would be able to know the products that perform well in the market. Teams will then result in areas with high demands by adjusting their strategies accordingly.
The other advantage is the possibility to predict the future trends. The predictive modeling will help the leader in projecting the market change. All these lead to a well-decided decision that can reduce risks.
Predictive Analytics in Marketing
Predictive analytics uses historic data to predict customer behavior. This acts as a blueprint of marketing strategies.
By studying the history of purchases, a company can identify its prospective customers. For example, a person buying sporting goods frequently, there are chances of sending personalized ads. Thus, the opportunity of making sales is maximized.
Furthermore, the effectiveness of a campaign can be measured by the firm. They can try two different methods and then find out which investment pays the best dividend. In this way, marketing budgets can be optimized, improving revenue.
Better Customer Experience
Personalization: Data science helps in creating experiences tailored down to each customer—understanding their tastes and needs from data generated on multiple channels. For example, recommendation systems on a streaming service could suggest shows based on your viewing history, thus keeping the customer engaged and loyal to the service, which then encourages paid subscriptions.
Another important use case of feedback or response surveys can be in identifying areas of improvement. Enterprises can analyze the data to make necessary modifications, assuring that customer satisfaction comes first.
Operational Efficiency and Cost Reduction
Operational efficiency can be enhanced by making use of data science to cut costs. Workflows analysis pinpoints where the components of the workflow are not efficient.
For instance, manufacturing companies can track how their machines are performing in real-time. This thus enables the planning of predictive maintenance to service the equipment before it actually breaks down. It thus minimizes the downtime that contributes to waste and money loss.
Supply chain analysis is another area wherein one might identify cost-efficient suppliers. More competitive pricing thus leads to higher profit margins for the company. These operational decisions foster a culture of constant enhancement via data-driven action.
Also Read :
- The Rise of Quantum Computing: What It Means for Technology
- The Future of Mobile Technology: Trends to Watch in 2024
- Blockchain Technology: Understanding Its Impact Beyond Cryptocurrencies
- LG Technology Ventures
- FAE Technology Quote