AI-Powered Predictive Maintenance in Mechanical Systems
Predictive maintenance has emerged as a game-changer in the field of mechanical systems, leveraging artificial intelligence (AI) to predict equipment failures before they occur. By analyzing data collected from sensors and historical performance, AI-powered predictive maintenance enables industries to optimize operations, reduce downtime, and save costs. This article explores the principles, benefits, applications, and challenges of integrating AI into predictive maintenance strategies.
What is Predictive Maintenance?
Predictive maintenance is a proactive approach that uses data analytics and machine learning to assess the condition of mechanical systems and predict when maintenance should be performed. Unlike reactive maintenance, which addresses issues after they occur, or preventive maintenance, which schedules repairs based on time intervals, predictive maintenance focuses on condition-based servicing.
The Role of AI in Predictive Maintenance
AI technologies enhance predictive maintenance by:
- Data Analysis: Processing vast amounts of sensor data to detect patterns and anomalies.
- Machine Learning Models: Building predictive algorithms that learn from historical data to improve accuracy.
- Real-Time Monitoring: Continuously assessing the health of machinery and providing actionable insights.
- Failure Prediction: Forecasting potential breakdowns based on early warning signs.
- Optimization: Recommending maintenance schedules to minimize disruptions and costs.
Key Benefits of AI-Powered Predictive Maintenance
- Reduced Downtime: Early detection of potential issues prevents unexpected equipment failures.
- Cost Savings: Minimizing unplanned repairs and extending the lifespan of mechanical components.
- Improved Safety: Identifying risks early reduces the likelihood of accidents.
- Efficiency Gains: Streamlining maintenance schedules enhances operational efficiency.
- Scalability: AI models can handle data from multiple machines, making it suitable for large-scale operations.
Applications in Mechanical Systems
- Manufacturing:
- Monitoring CNC machines, assembly lines, and robotics for wear and tear.
- Automotive:
- Predicting engine and transmission failures to reduce warranty claims and improve vehicle reliability.
- Aerospace:
- Analyzing aircraft engines and critical components to ensure flight safety.
- Energy Sector:
- Assessing turbines, compressors, and generators in power plants for optimal performance.
- Oil and Gas:
- Monitoring drilling rigs and pipelines for corrosion and mechanical stress.
AI Techniques Used in Predictive Maintenance
- Time Series Analysis: Evaluating trends in sensor data over time.
- Anomaly Detection: Identifying deviations from normal operating conditions.
- Classification Models: Categorizing equipment conditions as healthy, at risk, or critical.
- Regression Models: Predicting the remaining useful life (RUL) of components.
- Natural Language Processing (NLP): Analyzing maintenance logs for insights into recurring issues.
Challenges in Implementation
- Data Quality: Inaccurate or incomplete data can hinder AI model performance.
- High Initial Costs: Implementing AI-driven solutions requires significant investment.
- Integration Issues: Combining AI with legacy systems can be complex.
- Skill Gaps: Training personnel to work with AI technologies is essential.
- Cybersecurity Risks: Protecting sensitive data from breaches is a critical concern.
Future Trends
- Edge Computing: Processing data locally on devices to reduce latency and enhance decision-making.
- Digital Twins: Creating virtual replicas of mechanical systems for simulation and analysis.
- Autonomous Maintenance: Using AI to automate certain repair tasks without human intervention.
- Cloud Integration: Leveraging cloud platforms for centralized data storage and advanced analytics.
- Interoperability: Ensuring seamless communication between AI systems and industrial IoT devices.
Conclusion
AI-powered predictive maintenance is revolutionizing the way industries manage mechanical systems. By harnessing the power of AI, organizations can transition from reactive to proactive strategies, improving reliability, safety, and cost-effectiveness. While challenges remain, the continued evolution of AI technologies promises a future where maintenance is smarter, faster, and more efficient than ever before.
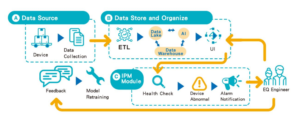
Also Read :
- Exploring New Materials for Space Exploration: An Engineering Perspective
- Hydrogen Fuel Cells: Engineering Cleaner Energy Systems
- The Impact of 3D Printing on Biomedical Engineering
- The Cutting Edge: How is Shaping Tomorrow’s Engineering
- MRI in Pediatric Neurosurgery: Unique Challenges