Artificial Intelligence (AI) has made remarkable strides in recent years, transforming industries from healthcare to finance, customer service to entertainment. However, AI’s effectiveness is only as strong as the data it learns from, and this brings us to a crucial issue: diversity. When data lacks inclusivity, AI models risk reinforcing existing biases, making unfair decisions, or excluding certain groups entirely. Inclusive data ensures that AI can serve everyone equitably, regardless of background, gender, race, or socioeconomic status. This article dives into why diversity is essential for AI, the risks of excluding it, and the steps we can take to create more inclusive, fair AI systems.
The Role of Data in Shaping AI
AI learns by analyzing vast amounts of data, detecting patterns, and making predictions based on those patterns. For example, a machine learning model trained to recognize faces will look at thousands (or even millions) of images to learn how to identify human features. However, if most of those images represent only certain demographic groups, the model might struggle—or even fail—when presented with faces from underrepresented groups. Thus, data diversity directly impacts the reliability, fairness, and overall accuracy of AI.
Real-World Implications of Biased Data
When AI is trained on biased or non-diverse data, it can lead to harmful real-world consequences. For instance, facial recognition systems have been shown to be less accurate for people with darker skin tones, leading to potential misidentifications or failures in security applications. Similarly, AI used in hiring can unintentionally favor certain groups if it’s trained on biased historical data. These examples highlight the importance of inclusive data in creating AI that serves everyone fairly.
How AI Bias Arises: The Data Problem
The lack of diversity in AI often stems from the data used to train these systems. When the data isn’t inclusive, AI models inherit the biases and limitations present in those datasets. There are several reasons why these biases occur:
- Historical Bias: Historical data often reflects the biases of society. For example, if a hiring dataset primarily contains men in certain roles, an AI model might inadvertently learn to favor male candidates.
- Sampling Bias: When datasets don’t accurately represent the population, sampling bias occurs. For instance, a medical AI trained mostly on data from white patients might fail to detect certain conditions that present differently in other ethnic groups.
- Confirmation Bias: AI models trained on datasets that reinforce existing stereotypes or expectations can inadvertently perpetuate these biases.
The Risks of Non-Inclusive AI
AI systems that are not trained with diverse data can have severe implications, particularly when they are deployed in sensitive areas like law enforcement, healthcare, and hiring. Here are some of the most pressing risks:
Discrimination and Exclusion
When AI models are biased, they risk excluding or discriminating against certain groups. For example, a loan approval model trained primarily on data from affluent neighborhoods might deny loans to applicants from lower-income areas, not due to their creditworthiness but due to biased training data.
Loss of Trust in AI Systems
For AI to be widely accepted, people must trust it. When AI shows signs of bias, it erodes that trust, making individuals skeptical of its fairness and effectiveness. If a facial recognition model misidentifies individuals from minority groups more frequently, public confidence in the technology diminishes, hindering AI adoption.
Reinforcement of Social Inequities
AI models trained on non-diverse data can inadvertently reinforce existing social inequities, perpetuating a cycle of disadvantage. For example, if an AI hiring model favors candidates from specific backgrounds, it limits opportunities for underrepresented groups, reinforcing inequity in the workforce.
The Business Case for Inclusive AI
Beyond the ethical imperatives, there are strong business reasons for making AI inclusive. Diverse AI not only enhances user experiences but also widens the customer base by appealing to a broader range of people. Here’s why inclusive AI makes good business sense:
Access to a Broader Market
By ensuring that AI models work effectively for all demographic groups, companies can reach a wider customer base. For instance, if a virtual assistant understands and responds accurately to users with different accents and dialects, it becomes more accessible and useful to a global audience.
Improved Innovation
Diverse perspectives fuel creativity and innovation. When AI is trained on a broader range of data, it can solve problems in new and unexpected ways, fostering innovation. Inclusive AI also helps avoid the pitfalls of groupthink, leading to more robust, well-rounded solutions.
Competitive Advantage
Companies that prioritize inclusivity in their AI systems can differentiate themselves as leaders in ethical AI. As consumers become more aware of the importance of ethical and inclusive technology, businesses that emphasize diversity in their AI strategies gain a competitive edge.
How to Build AI with Inclusive Data
Creating inclusive AI requires a concerted effort at every stage of the development process, from data collection to model deployment. Here are some key steps to ensure data diversity:
Collecting Diverse Datasets
The first step to inclusivity is ensuring that the training data reflects the diversity of the real world. This involves collecting data from different demographic groups, including variations in age, gender, race, socioeconomic background, and more. In fields like healthcare, it’s critical to ensure that datasets include samples from diverse ethnic and geographic backgrounds.
Auditing and Testing for Bias
Regular audits of AI models are essential to identify and address biases. Bias detection tools can help evaluate the performance of AI systems across different demographic groups, pinpointing areas where the model may be unfair or inaccurate.
Involving Diverse Teams in AI Development
Diverse development teams bring different perspectives and insights, helping to spot potential biases that might otherwise go unnoticed. When people from varied backgrounds contribute to AI projects, they are more likely to consider inclusivity in design and functionality, leading to fairer AI systems.
Continuous Monitoring and Feedback
AI is not a one-and-done solution. Once deployed, AI models should be continuously monitored to ensure they perform fairly across all groups. Feedback from users, especially from underrepresented communities, can help identify issues that may require re-training or model adjustments.
Real-World Examples of Inclusive AI Initiatives
Some organizations are taking steps to build more inclusive AI, setting examples for the industry to follow:
- Google’s Inclusive Images Initiative: Google has worked on reducing bias in image recognition by expanding its datasets to include images from various countries, cultures, and demographics.
- IBM’s Fairness 360 Toolkit: IBM provides tools that allow developers to test their AI models for fairness, enabling companies to detect and correct biases before deployment.
- Microsoft’s AI for Accessibility: Microsoft is developing AI solutions specifically designed to be accessible for individuals with disabilities, ensuring that AI systems are usable and inclusive for all.
Ethical and Regulatory Considerations for Inclusive AI
As awareness of AI bias grows, governments and regulatory bodies are beginning to introduce guidelines to promote fairness in AI. Regulations such as the EU’s GDPR and the U.S. Federal Trade Commission’s (FTC) guidelines on AI ethics emphasize transparency, accountability, and fairness in AI practices. Businesses should stay informed of these regulations to ensure their AI systems comply with ethical standards and foster public trust.
Conclusion: Embracing Diversity for a Fairer Future in AI
The case for inclusive data in AI is not only a moral imperative but also a business advantage. AI systems that understand and fairly represent all demographics are better equipped to serve society’s diverse needs. By focusing on inclusive data collection, regular bias audits, and diverse development teams, companies can create AI systems that are fair, ethical, and effective. Embracing diversity in AI isn’t just about technology; it’s about building a future where everyone benefits from the advancements AI has to offer.
FAQs
- What is the importance of diversity in AI data?
Diversity in AI data helps to ensure that AI models are fair, accurate, and inclusive, reducing the risk of biased decisions that can negatively affect certain demographic groups. - How does biased data affect AI outcomes?
Biased data can lead AI models to make unfair or discriminatory decisions, reinforcing societal inequities and creating a lack of trust in AI systems. - What are some examples of inclusive AI practices?
Examples include Google’s Inclusive Images Initiative, IBM’s Fairness 360 Toolkit, and Microsoft’s AI for Accessibility, which aim to make AI fairer and more accessible. - Can AI models be re-trained to reduce bias?
Yes, AI models can be re-trained using more diverse and representative data to reduce biases and improve fairness in outcomes. - How can businesses ensure their AI systems are inclusive?
Businesses can ensure inclusivity by collecting diverse datasets, regularly auditing for bias, involving diverse development teams, and adhering to ethical and regulatory standards.
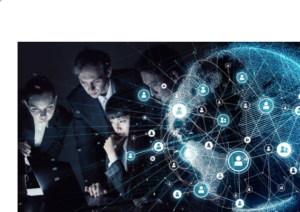
Also Read :