The evolution of artificial intelligence (AI) is one of the most remarkable technological journeys of our time — and at the core of this transformation lies relentless progress in engineering. From revolutionary hardware to sophisticated software systems, groundbreaking engineering advances are propelling AI beyond theoretical possibilities into real-world applications that are changing industries, societies, and everyday life.
In this article, we explore how engineering is breaking boundaries and fueling the next generation of AI innovation.
Engineering the Foundations of AI Power
Before AI could become what it is today, the infrastructure supporting it had to be engineered. The design and development of powerful computational hardware laid the foundation for AI’s explosive growth.
Hardware Innovations Empowering AI
- Graphics Processing Units (GPUs): Originally designed for rendering graphics, GPUs revolutionized AI by enabling rapid, parallel processing necessary for machine learning and deep learning tasks.
- Tensor Processing Units (TPUs): Engineered specifically for AI, TPUs deliver enhanced speed and efficiency for neural network training and inference.
- Quantum Computing: Though still emerging, quantum technology represents a future frontier, offering the potential to solve AI problems far beyond the capabilities of traditional computers.
- Neuromorphic Chips: Inspired by the human brain’s structure, these chips are engineered to improve energy efficiency and processing power for AI tasks, paving the way for more autonomous and adaptive systems.
Each leap in hardware engineering removes previous performance limitations, allowing AI to grow smarter, faster, and more capable.
Revolutionizing Data Systems: Engineering the Lifeblood of AI
AI thrives on data — but without advanced data engineering, that raw information would be chaotic and unusable.
Key Data Engineering Contributions:
- Big Data Architectures: Systems like Hadoop, Spark, and cloud-native solutions have been engineered to handle petabytes of structured and unstructured data.
- Real-Time Processing Pipelines: Technologies such as Kafka and Flink enable AI systems to process information as it arrives, supporting applications like autonomous driving and fraud detection.
- Data Privacy Engineering: Solutions like federated learning and differential privacy protect sensitive information while maintaining AI’s learning capabilities.
- Synthetic Data Generation: Engineers create artificial datasets to train AI when real-world data is scarce or privacy concerns are high.
Data engineering breakthroughs ensure AI models are fueled by clean, comprehensive, and secure information streams.
Pushing Software Frontiers: Engineering Smarter AI Systems
Software engineering is the creative heart behind AI’s logic and learning. As AI algorithms grow more complex, engineers are developing new tools and frameworks to manage this complexity effectively.
Advances in Software Engineering for AI:
- Automated Machine Learning (AutoML): Engineers automate the design and optimization of machine learning models, making AI development more accessible and faster.
- MLOps Platforms: By borrowing concepts from DevOps, MLOps streamlines the deployment, monitoring, and scaling of AI models in production environments.
- Transfer Learning Techniques: Engineers enable AI systems to transfer knowledge across different tasks, drastically reducing the need for enormous datasets and training time.
- Explainable AI (XAI): Tools that reveal how AI models make decisions help to build trust and allow humans to understand complex algorithmic behaviors.
Software engineering ensures that AI doesn’t just grow in intelligence but also becomes more manageable, explainable, and ethically aligned.
Robotics: Where AI Meets Physical Engineering
Robotics showcases one of the most tangible intersections between AI and engineering. Mechanical, electrical, and computer engineers collaborate to create machines capable of sensing, thinking, and acting autonomously.
Engineering Contributions to AI Robotics:
- Sensor Fusion: Engineers integrate inputs from multiple sensors (cameras, LIDAR, sonar) to create AI-powered perception systems.
- Intelligent Motion Planning: Advanced algorithms enable robots to navigate complex environments, avoid obstacles, and optimize movement.
- Human-Robot Interaction (HRI): Engineers design systems that allow robots to work safely and intuitively alongside humans in fields like healthcare, manufacturing, and customer service.
Through multidisciplinary engineering, robots are becoming more intelligent, capable, and socially aware.
Engineering Ethical and Responsible AI
As AI systems take on critical roles in decision-making, ensuring ethical behavior and fairness becomes a paramount engineering challenge.
Solutions for Ethical AI Engineering:
- Bias Mitigation Tools: Engineers develop methods to detect and correct biases in AI datasets and algorithms.
- Auditability Frameworks: Systems are designed to track and explain AI decision-making processes for accountability.
- Ethical AI Standards: Engineers collaborate with policymakers to create technical standards that promote fairness, transparency, and user rights.
By embedding ethical principles into system designs, engineers ensure that AI technologies serve humanity responsibly and equitably.
Sustainability: Engineering for a Greener AI Future
The rapid growth of AI has raised environmental concerns, particularly related to energy consumption during model training and deployment. Engineering solutions are vital for making AI more sustainable.
Innovations in Sustainable AI Engineering:
- Energy-Efficient Algorithms: Techniques like model pruning, knowledge distillation, and quantization reduce computational requirements.
- Green Data Centers: Engineers design facilities that use renewable energy, advanced cooling systems, and AI itself to optimize energy use.
- Hardware Optimization: Developing chips that consume less power while maintaining performance addresses the environmental footprint of AI hardware.
Engineering sustainable practices into AI’s lifecycle is critical for long-term, responsible technological advancement.
Future Engineering Breakthroughs Shaping AI
As the partnership between engineering and AI continues to deepen, several exciting frontiers promise to further revolutionize the field.
Emerging Trends:
- Brain-Computer Interfaces (BCIs): Engineers are developing systems that allow direct communication between AI systems and the human brain.
- Bio-Inspired Computing: Mimicking natural intelligence, engineers are creating systems that learn and adapt more organically.
- Swarm Intelligence: Engineering AI systems that cooperate like flocks of birds or schools of fish, offering new models for problem-solving.
- Self-Healing Systems: Future AI models may autonomously detect and fix issues without human intervention, thanks to sophisticated engineering designs.
These breakthroughs will not just evolve AI; they will redefine how we interact with technology, work, and even think.
Conclusion: Engineering — The Force Behind AI’s Next Leap
The evolution of artificial intelligence would not be possible without the relentless advances of engineering. Every leap forward — whether in computational power, data handling, ethical governance, or sustainable practices — has been engineered with vision, precision, and innovation.
As AI continues to break boundaries, it will be the engineers — the architects of our intelligent future — who pave the way. By fusing creativity, technical expertise, and ethical responsibility, engineering will continue to drive AI to new horizons, transforming how we live, work, and connect with the world.
In the end, AI’s future is limited only by the imagination and ingenuity of the engineers who dare to build it.
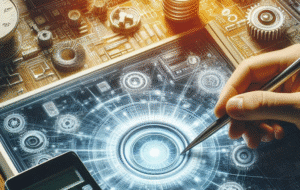
Also Read :